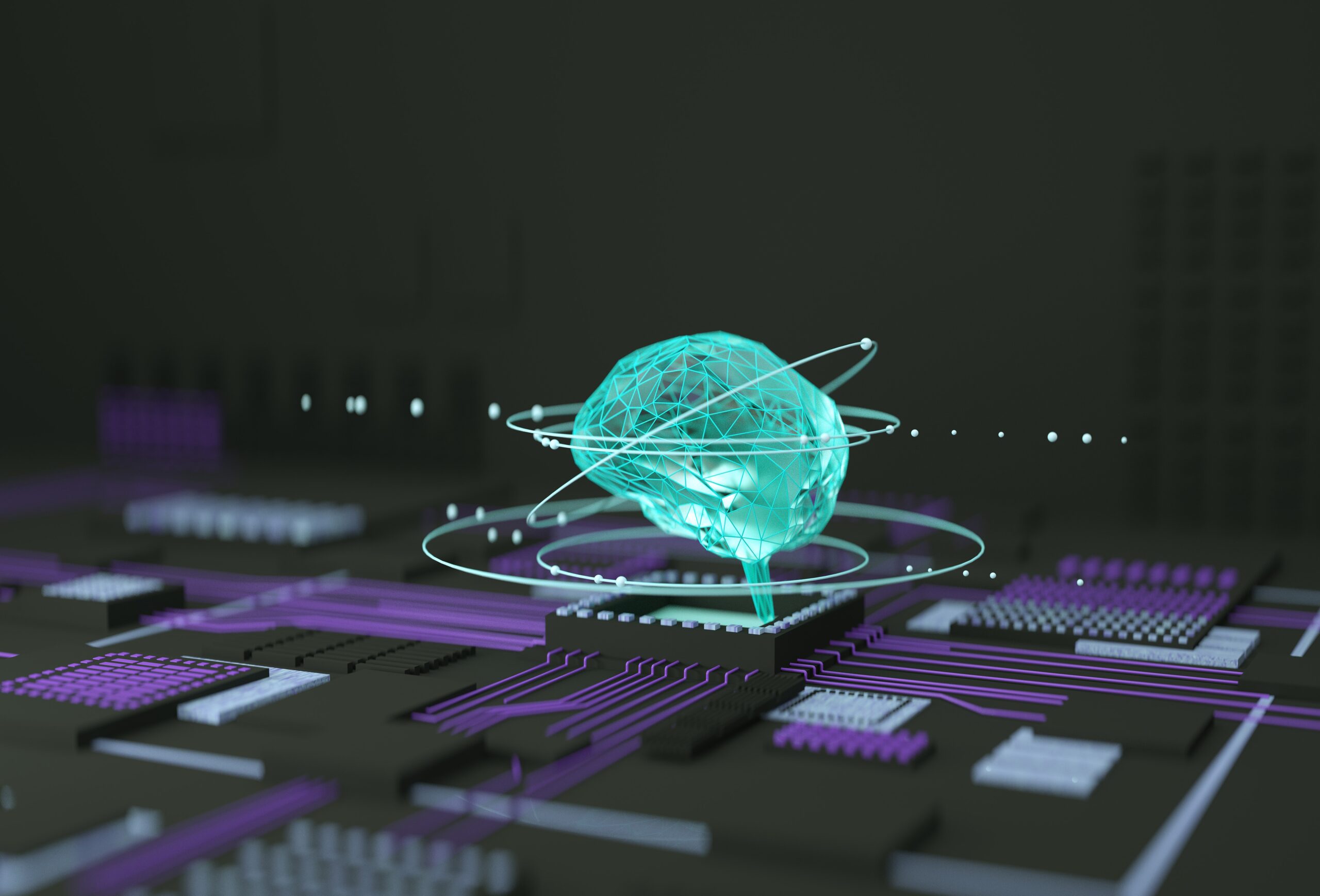
Unlocking the Potential of Large Language Models in Materials Science: A Revolutionary Shift
In the context of a rapidly evolving technological
landscape, the use of large language models (LLMs) is revolutionising the way
we approach complex tasks. These sophisticated models, such as the Generative
Pretrained Transformer (GPT), Meta’s LLaMA or Mistral 7B are not only powerful
linguistic tools but also potentially relevant assets in various scientific
disciplines.
In the field of materials science, where the search for
novel biomaterials holds significant promise, the fusion of natural language
processing (NLP) with deep learning techniques has led to a new era of
efficiency and innovation. One such application is materials language
processing (MLP), a cutting-edge approach that aims to facilitate materials
science research by automating the extraction of structured data from vast
repositories of research papers and literature content.
Although MLP has the potential to revolutionise materials
science research, it has faced practical challenges, including the complexity
of model architectures, the need for extensive fine-tuning, and the lack of
human-labelled datasets. However, recent developments, such as those described
in the work of Jaewoong Choi et al. [1], have enabled a revolutionary shift
leveraging the power of in-context learning and prompt engineering of LLMs. In
this research, Jaewoong Choi employed a GPT-based approach to filter papers
relevant to battery materials using two classification categories:
“battery” or “non-battery.” This was achieved through LLM
prompt engineering and the use of a limited number of training data. Figure 1
illustrates an input example of the developed GPT-enabled zero-shot text
classification approach, i.e., without fine-tuning the model with
human-labelled data.
This approach has demonstrated the capacity to achieve high
performance and effectiveness in a wide range of tasks, including text
classification, named entity recognition, and extractive question answering
across different classes of materials. The versatility of generative models
extends beyond performance metrics, as they serve as useful tools for error
detection, identification of inaccurate annotations, and refinement of
datasets. This has resulted in materials scientists being able to perform complex
MLP tasks with confidence, even in the absence of a wide domain-specific
expertise.
In essence, the convergence of large language models and
materials science represents a paradigm shift in scientific methodology. The
capacity of these transformative technologies to identify patterns and extract
insights from vast volumes of textual data not only enhances human capabilities
but also redefines the paradigm of scientific exploration.
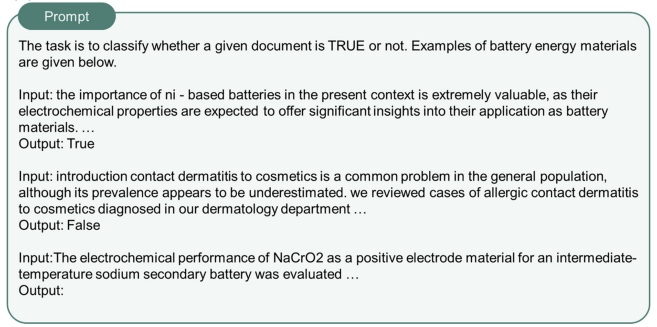
Author: Miguel Rodríguez Ortega, Jan Rodríguez Miret
Links
[1] Choi, J., Lee, B. Accelerating materials language processing with large language models. Commun Mater 5, 13 (2024). https://doi.org/10.1038/s43246-024-00449-9
Keywords
Material science, Large Language Models, GPT, Material Language Processing, Text Mining