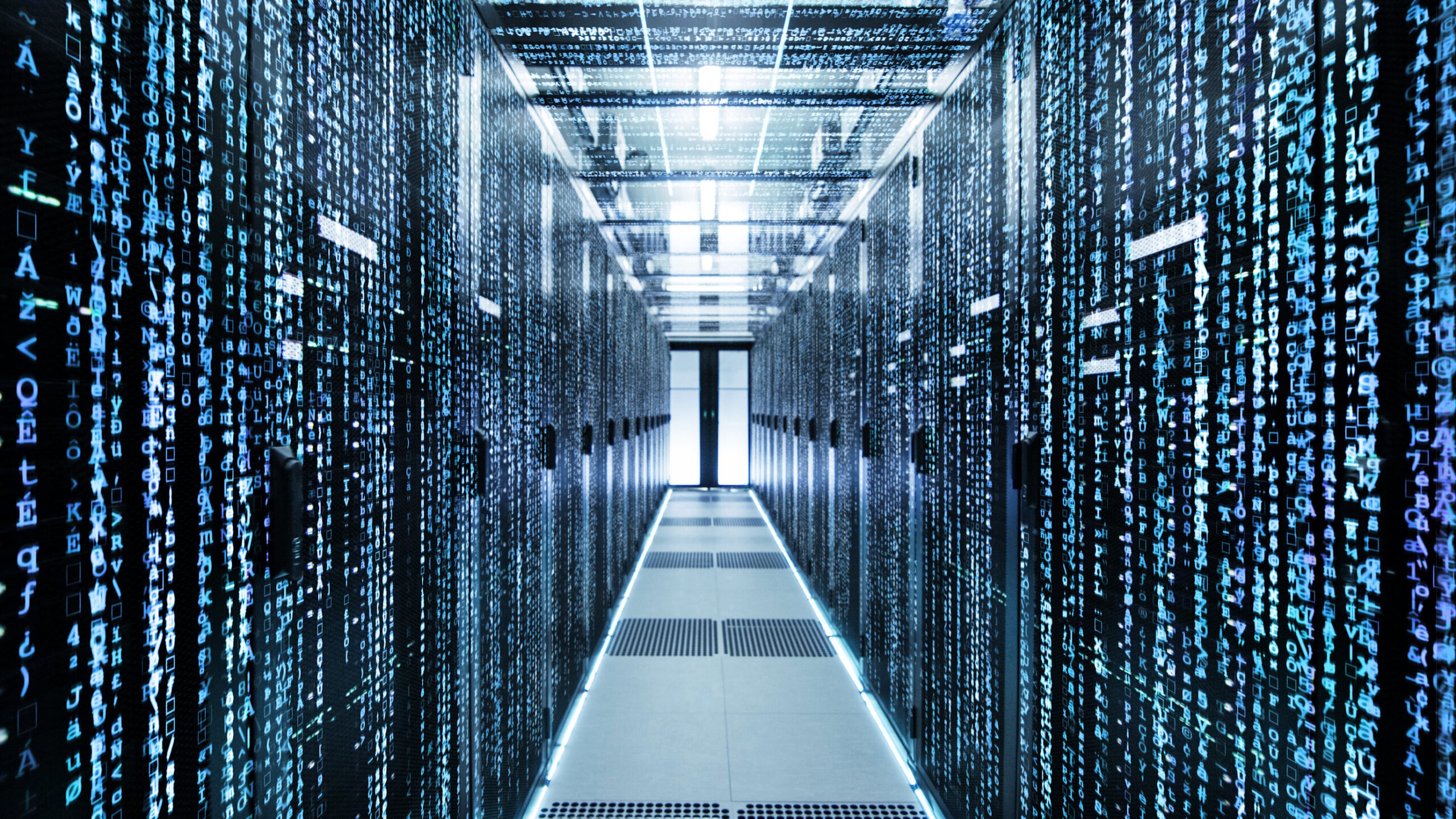
Application of Text Mining in Materials Science
Given the pervasive influence of text mining on various societal
domains, the scientific domain in particular is witnessing an increasing
emphasis on data acquisition. In the realm of materials science, an increasing
demand exists for the extraction of knowledge, encompassing physical properties
and synthesis processes of materials from a substantial volume of scientific
papers. This field presents a unique challenge due to the inherent
heterogeneity resulting from the different classes of materials studied and the
range of material properties under investigation. As a result, the data
generated spans a wide range of scales, in the form of numerical data, textual
information or image-based content, requiring sophisticated quantitative
interpretation. Using techniques borrowed from natural language processing,
there is a compelling opportunity to automatically process and organise
scientific literature across multiple fields, unlocking and generating the rich
datasets essential for advances in data science and machine learning.
A review of advances and methodologies in
natural language processing and text mining as applied to the materials science
literature is presented in the work of Elsa A et al. [1]. They highlight the
potential to extract valuable information beyond the textual content found in
figures and tables within scholarly articles. This exploration is motivated by
various objectives, including data collection, hypothesis development, and
discerning trends within and across fields. The work delves into illustrative
examples and outlines current and emerging natural language processing methods,
highlighting their applications in the context of materials science. It also
examines the challenges posed by natural language processing and the
intricacies of data in materials science, and provides insights into possible
directions for future developments.
Another example of the application of text mining methods, in this
case in the field of inorganic materials science, is presented in the work of
Kuniyoshi et al [2]. In this work, they introduce a large-scale Natural
Language Processing (NLP) pipeline designed to extract material names and
properties from the materials science literature to facilitate search and
retrieval of results in the field. To achieve this goal, a label definition is
proposed for the extraction of material names and properties, resulting in the
creation of a corpus of 836 annotated paragraphs extracted from 301 papers.
This corpus serves as training data for a named entity recognition (NER) model.
Experimental results demonstrate the effectiveness of the NER model, which
achieves successful extraction with a micro-F1 score of 78.1%. To further
demonstrate the usefulness of the approach, a comprehensive evaluation is
performed on a real-world automatically annotated corpus, using the trained NER
model on 12,895 materials science papers.
Authors:
Miguel Rodríguez, Jan Rodríguez
References
[1] Elsa A. Olivetti ; Jacqueline M. Cole ; Edward Kim ; Olga Kononova; Gerbrand Ceder;
Thomas Yong-Jin Han ; Anna M.
Hiszpanski. “Data-driven materials research enabled by natural language
processing and information extraction”. Available from: https://dspace.mit.edu/handle/1721.1/142580
[2] Fusataka
Kuniyoshi, Jun Ozawa
& Makoto Miwa,
“Analyzing Research Trends in Inorganic Materials Literature Using NLP”.
Available from: https://arxiv.org/abs/2106.14157
Keywords
Text Mining, Materials, Natural Language Processing, Named Entity Recognition