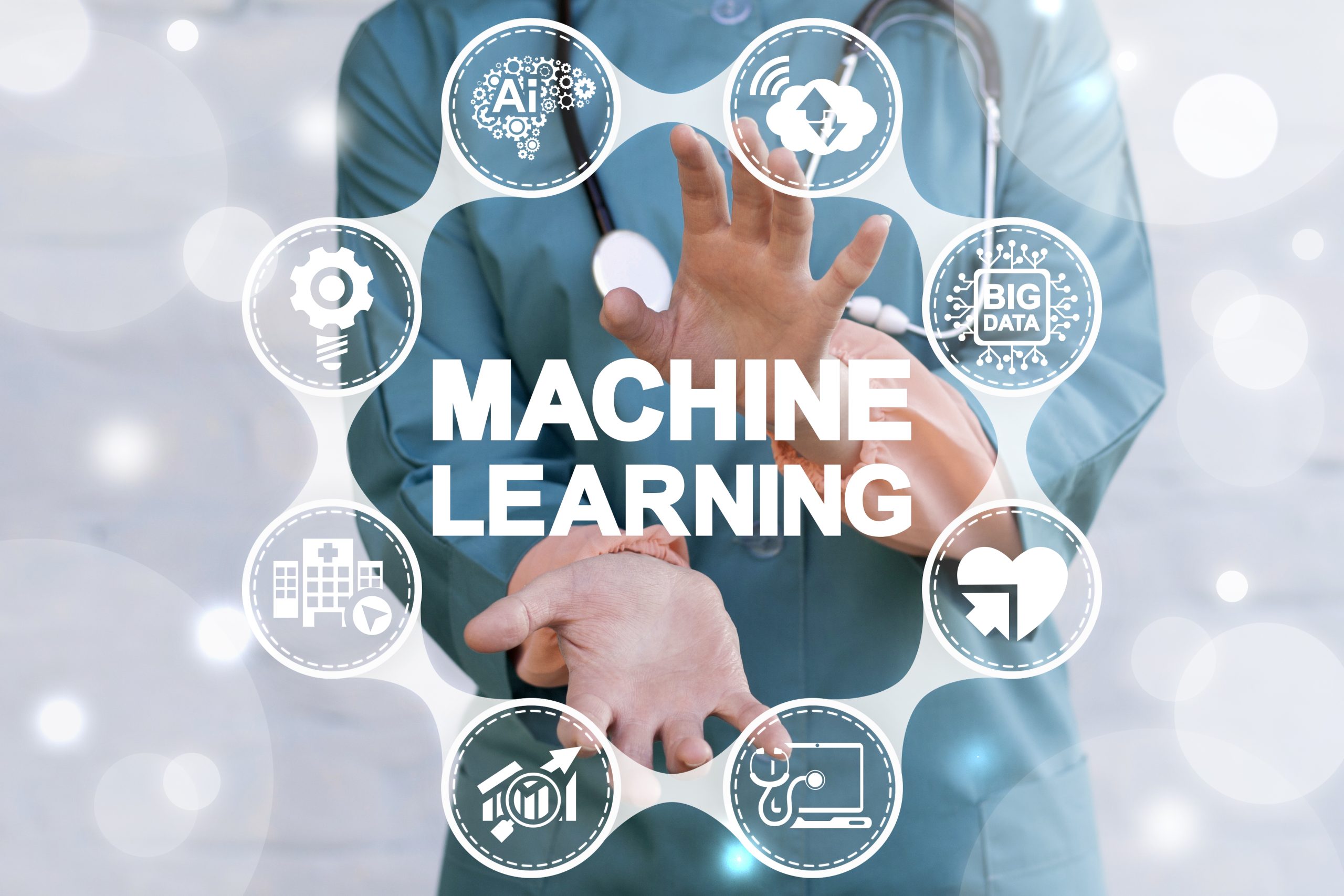
Machine learning for formulation of polymer microparticle-based long-acting injectables
Polymeric microparticles have become increasingly popular for drug delivery systems, particularly for long-acting injectables (LAI). One of the key features of these systems is their ability to achieve prolonged, controlled release of drugs over a period of weeks to months. This is particularly useful for compounds with poor water solubility, rapid clearance, or a short biological half-life. Importantly, by maintaining an optimal drug concentration for an extended time, LAI can improve drug efficacy while reducing the so-called dosage form index, the ratio between maximal and minimal drug concentrations achieved during a dose cycle. This can also minimize drug side effects by reducing peak dose. At the same time, the total amount of drug required can be lower, while maintaining—or even improving—efficacy, which can help reduce the cost of treatment. In this way, LAI are well-suited for delivering biologic therapies, such as protein-based drugs, or other drugs that need to be injected to avoid first-pass liver metabolism. Further, by eliminating the need for frequent dosing, LAI can minimize the risk of errors and reduce the burden of treatment on patients, thereby improving adherence to a therapeutic regime, even for drugs that could be delivered orally.
However, the development of microparticle drug formulations for use as LAI is particularly challenging. First, encapsulation can be difficult, because drug-polymer interactions based on the physico-chemical properties of both components drive compatibility. While there is a relatively limited range of Generally Recognized as Safe (GRAS) polymers available, each polymer actually has a range of variable properties, for example due to differences in molecular weight. When combined with the high diversity of drugs, the overall problem area is very large. Further, there are also many possible release mechanisms that are influenced by the properties of both polymer and drug. All of these factors combined result in a development process driven primarily by trial-and-error and requiring a great deal of experimental validation.
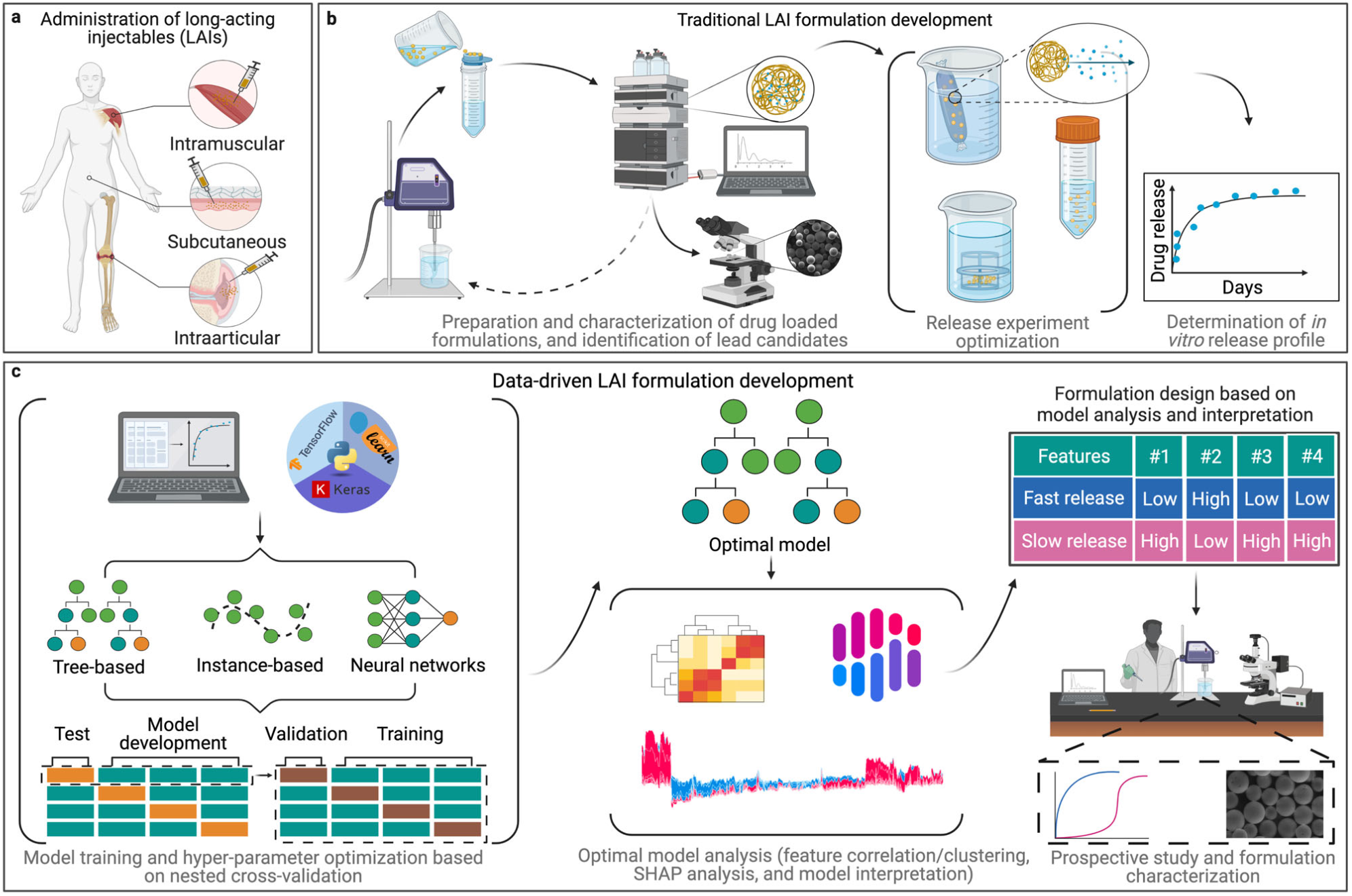
Figure 1: A) Examples of LAI delivery. B) Traditional workflow for preparing a polymer microparticle-based LAI formulation. C) Potential ML and data-driven workflow. Re-used under CC-BY 4.0 license from Bannigan, P., Bao, Z., Hickman, R.J. et al. Machine learning models to accelerate the design of polymeric long-acting injectables. Nat Commun 14, 35 (2023). Copyright © 2023 The Authors..
Potential of machine learning
In this context, machine learning (ML) has the potential to revolutionize the development of LAI for drug delivery. ML may be able to predict drug release profiles, based on a combination of drug and polymer molecular and physico-chemical properties. This can dramatically reduce the problem space, leading to savings in time, money, and resources associated with LAI formulation development.
In their recent study, Bannigan et al. (2023) explored this potential of ML to guide the formulation of LAI by testing a range of ML algorithms and establishing the possibility of data-driven LAI formulation. The authors focused on polymeric microparticles for LAI and collected a dataset of papers with drug, polymer, and release kinetics, comprising 181 drug release profiles for 43 unique drug-polymer combinations. They identified 17 unique descriptors, including molecular and physico-chemical properties of both drug and polymer, and then trained and tested a number of different ML models on their ability to predict experimental drug release. This aspect of the study showed that the light gradient boosting machine (LGBM) ML framework performed best at predicting fractional release over time. Further, the Shapley additive explanation (SHAP) analysis indicated that, for the LGBM model, the drug molecular weight (Mw) and polymer Mw were the two most important inputs affecting fractional release (after time).
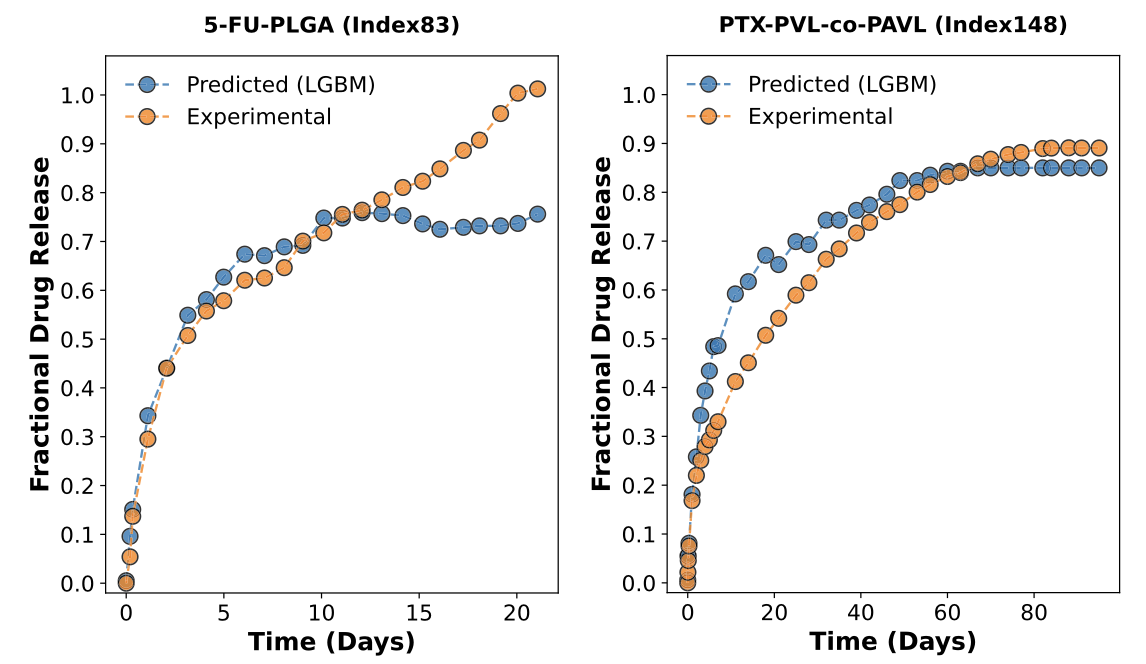
Figure 2: Examples of LGBM-predicted fractional release curves (blue) versus experimental data for fluorouracil-loaded PLGA microparticles (5- FU-PLGA) and paclitaxel-loaded PVL-co-PAVL cross-linked cylinders (PTX-PVL-co-PAVL). Re-used under CC-BY 4.0 license from Bannigan, P., Bao, Z., Hickman, R.J. et al. Machine learning models to accelerate the design of polymeric long-acting injectables. Nat Commun 14, 35 (2023). Copyright © 2023 The Authors.
Next, the authors set out to test the model predictions for encapsulation of salicylic acid (a pain killer) in a fast-release LAI and Olaparib (a chemotherapeutic) in a slow-release LAI. Importantly, these drugs were not present in the data set used to train the model. For the fast-release LAI, they utilized PLGA with a molecular weight of 10 kDa to prepare the microparticles, while for the slow-release LAI the molecular weight of the PLGA was 50 kDa. In both cases, the microparticles were prepared using conventional oil-in-water emulsification. Then, the authors evaluated the in vitro drug release profiles and compared them to the predictions of the ML model. For the case of the ML-guided fast release LAI, the experimental data was in very good agreement with model predictions. Meanwhile, for the case of the slow-release LAI, the experimental data matched the predictions well for the first 15 days, after which the actual release increased significantly. The authors attribute this to hydrolytic degradation of the PLGA polymer under the in vitro test conditions, which would result in faster drug release. They note that that data regarding hydrolytic degradation must be added in future ML model development for slow-release LAI.
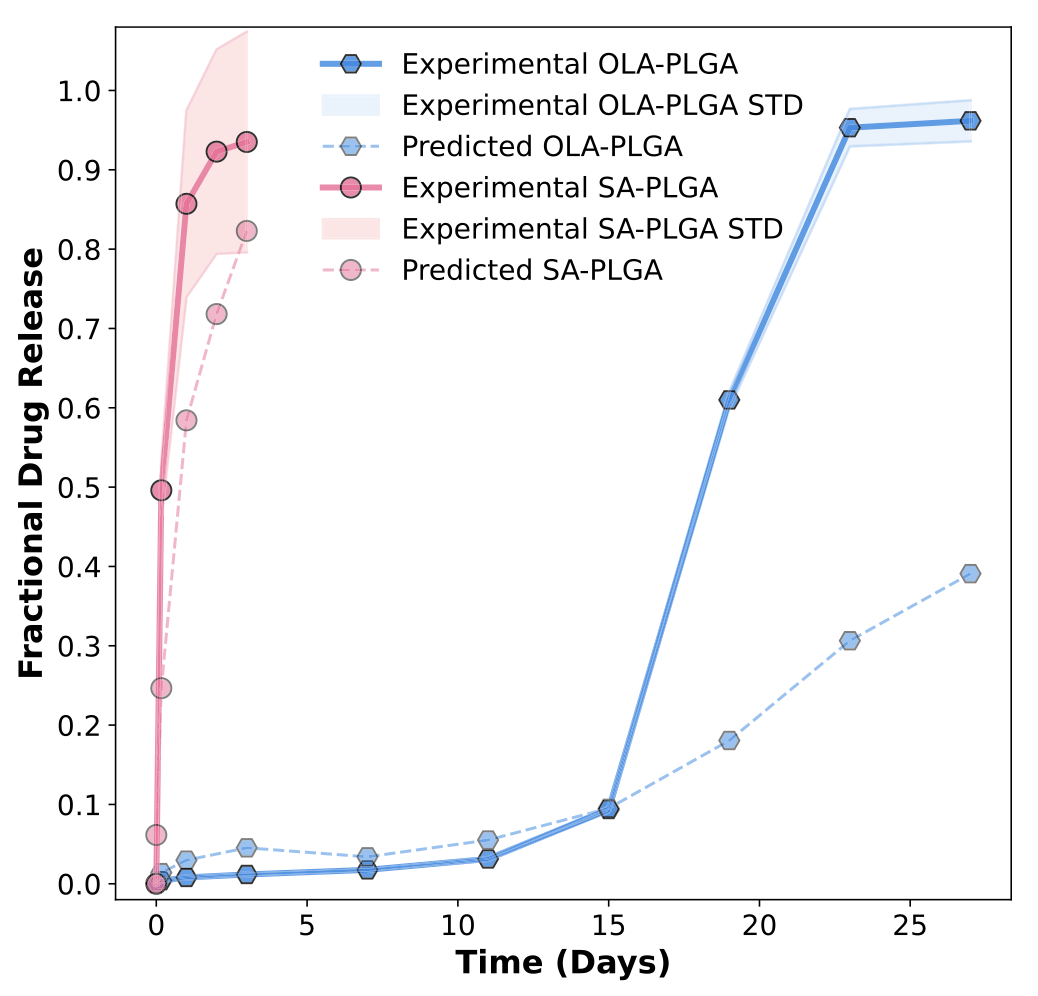
Figure 3: Comparison between experimental data (solid lines) for the fast (red) and slow (blue) release LAI formulated based on the trained LGBM model and the predicted fractional release curves (dashed lines). Re-used under CC-BY 4.0 license from Bannigan, P., Bao, Z., Hickman, R.J. et al. Machine learning models to accelerate the design of polymeric long-acting injectables. Nat Commun 14, 35 (2023). Copyright © 2023 The Authors.
Conclusions and future directions
Overall, the work of Bannigan et al. (2023) shows that ML approaches hold promise for LAI development. With proper choice of framework, ML models can predict drug release profiles from polymer microparticle-based LAI and further SHAP analysis can identify key formulation parameters. Meanwhile, the study’s prospective experiment, where the authors used ML guidance to formulate two LAI for drugs that were not in the training data, demonstrated the potential for speeding up LAI development. However, the study also highlighted the crucial role played by the dataset used to train the ML models. Here, the hydrolytic degradation of the polymeric matrix was underestimated.
Nevertheless, the study illustrates the potential of ML to guide the development of LAI and provide a data-driven alternative to traditional trial-and-error formulation methods. To fulfil this promise, the continued development of ML frameworks needs to go hand-in-hand with the collection and curation of larger and more diverse datasets that better reflect drug release conditions. A key element of the work involved collecting data from the literature and then curating and collating it into a dataset that could then be made machine-readable. Limitations in this training dataset were reflected in limitations of the trained ML model. In the future, we hope that the BIOMATDB database can help provide easier access to biomaterial properties and data needed for improved training of ML models, resulting in better predictions.
Authors: Peter Sobolewski
Reference
Bannigan, P., Bao, Z., Hickman, R.J. et al. Machine learning models to accelerate the design of polymeric long-acting injectables. Nat Commun14, 35 (2023). https://doi.org/10.1038/s41467-022-35343-w